Machine learning for salivary gland ultrasound scoring
A machine learning apporach to salivary gland ultrasound scoring in order to eliminate inter-reader variability and provide more exact prognostication.
Project Overview
Sjögren’s syndrome (SjS) is a systemic autoimmune diseases (SAD) that causes the dysfunction of the exocrine glands (mainly the salivary and lacrimal glands) with patients often showing persistent dryness of the mouth and eyes [1, 2]. According to estimations, two to four million persons in the United States have SjS; however, only one million have been diagnosed, likely due to the nonspecific diagnostic guidelines and the heterogeneity nature of the disease [3].
The current standard of care of the client is to perform at least baseline salivary gland ultrasounds (of the submandibular and parotid glands) in SjS patients. For some higher risk individuals, regularly scheduled salivary gland ultrasounds are performed. The problem arises within the current OMERACT ultrasound grading system, which requires subjective opinions and lacks nuance. As a result, a machine learning approach is proposed to eliminate inter-reader variability and to strive to provide more exact prognostication.
Team Picture
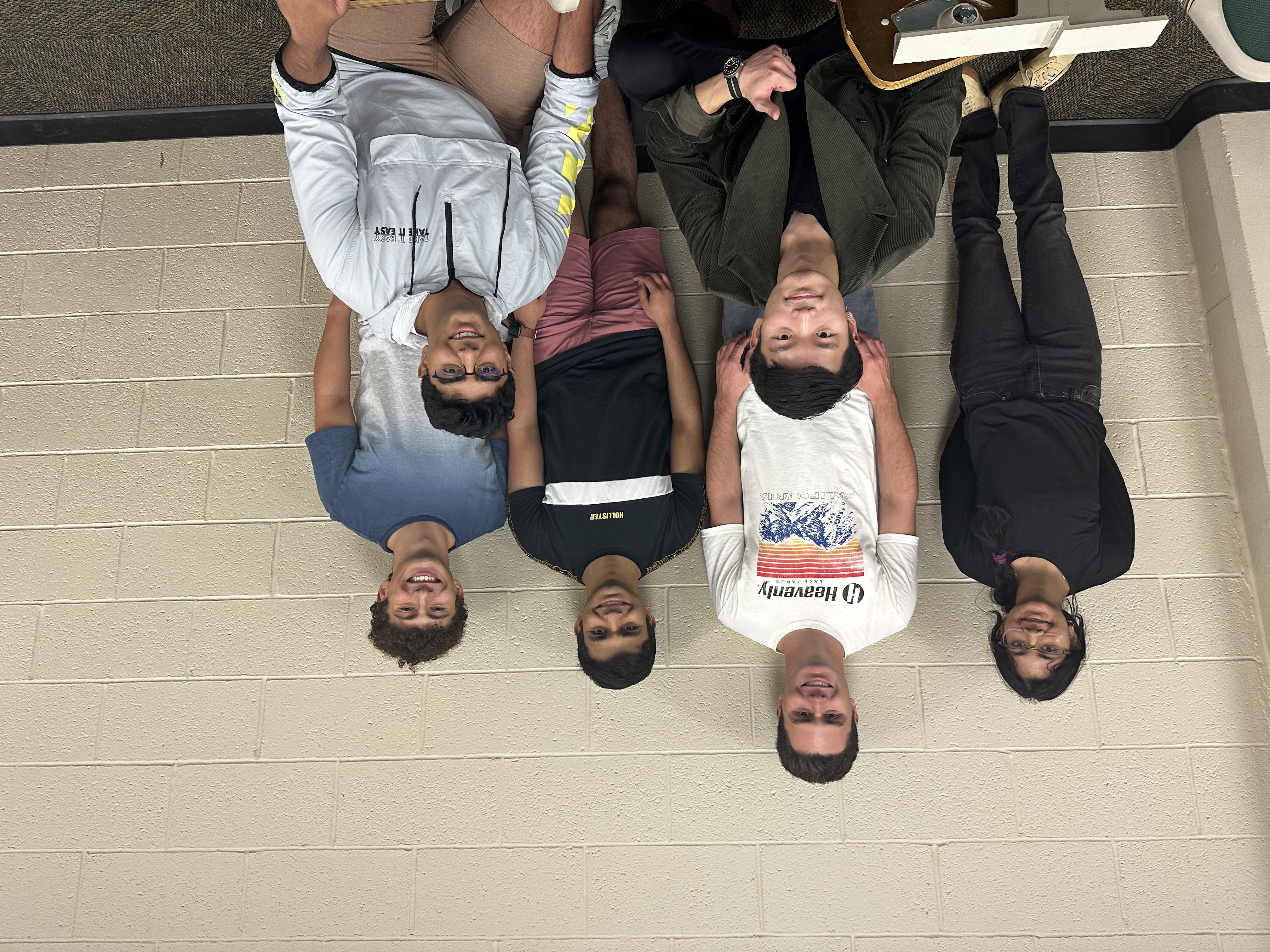
Files
- Final Notebook (December 13, 2023)
- Final Report (December 13, 2023)
- Final Poster Presentation (December 7, 2023)
- Preliminary Report (October 11, 2023)
- Preliminary Presentation (October 6, 2023)
- PDS (September 22, 2023)
Contact Information
Team Members
- Richard Yang - Team Leader
- Yousef Gadalla - Communicator
- Brandon Drew - BSAC
- Dhruv Nadkarni - Co-BWIG
- Siya Mahajan - Co-BWIG
- Aran Viswanath - BPAG
Advisor and Client
- Dr. Cameron Casey - Advisor
- Dr. Sara McCoy - Client